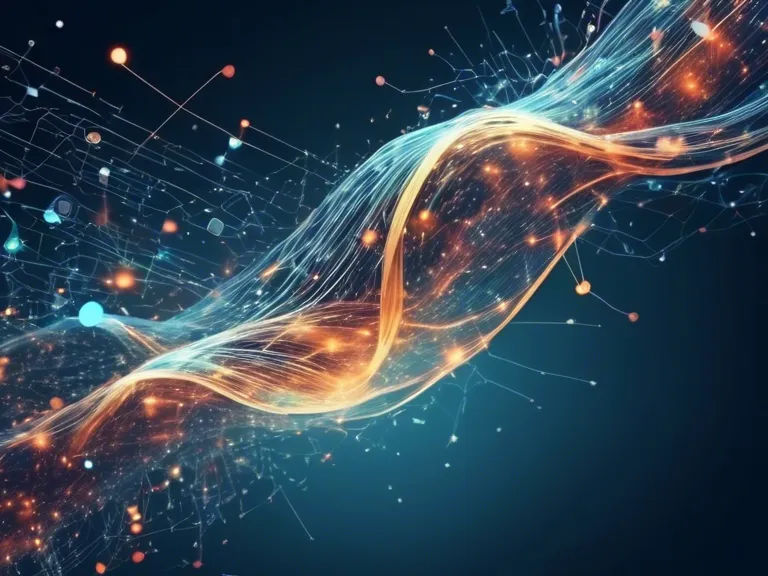
Introduction
Machine learning has revolutionized various industries by enabling computers to learn from data and make intelligent decisions without being explicitly programmed. To accelerate success in machine learning projects, organizations need to adopt effective strategies for rapid progress. In this article, we will explore key strategies that can help accelerate success in machine learning endeavors.
Setting Clear Goals and Objectives
One of the crucial steps in accelerating success in machine learning projects is setting clear goals and objectives. Define what success looks like for the project, identify key performance indicators (KPIs) that align with the business objectives, and establish a roadmap for achieving those goals.
Data Quality and Preparation
High-quality data is the foundation of successful machine learning models. Ensure that your data is clean, relevant, and properly labeled. Invest time in data preprocessing, feature engineering, and data augmentation to improve the quality of the dataset, which will ultimately lead to better model performance.
Choosing the Right Algorithms
Selecting the appropriate machine learning algorithms is essential for achieving rapid progress. Consider the nature of your data, the problem you are trying to solve, and the computational resources available when choosing algorithms. Experiment with a variety of algorithms to determine which ones perform best for your specific use case.
Continuous Learning and Experimentation
Machine learning is an iterative process that requires continuous learning and experimentation. Encourage a culture of experimentation within your team, where different approaches are tested, and learnings are shared. Keep track of model performance metrics and iterate on your models to improve accuracy and efficiency.
Leveraging Automation and Tools
Automation and tools can significantly speed up the machine learning workflow. Utilize tools for data preprocessing, model training, hyperparameter tuning, and model deployment to streamline the process and reduce manual effort. Adopting automation can help accelerate the development and deployment of machine learning models.
Collaboration and Knowledge Sharing
Promote collaboration and knowledge sharing among team members working on machine learning projects. Encourage cross-functional teams where data scientists, engineers, and domain experts work together to leverage their respective expertise. Sharing knowledge and best practices can lead to innovative solutions and accelerate progress.
Monitoring and Evaluation
Monitoring model performance and evaluating its impact on business outcomes is crucial for accelerating success in machine learning projects. Implement monitoring tools to track model performance in real-time, identify issues early, and make necessary adjustments to improve model performance continuously.
Conclusion
Accelerating success in machine learning projects requires a combination of strategic planning, quality data, appropriate algorithms, continuous learning, automation, collaboration, and monitoring. By implementing these strategies effectively, organizations can achieve rapid progress, drive innovation, and unlock the full potential of machine learning technologies. Embrace a data-driven culture, prioritize experimentation, and leverage the power of machine learning to accelerate success in your endeavors.